Ever wonder how Netflix just seems to know what you want to watch next? It’s not magic—it’s artificial intelligence at work. Recommendation engines use AI and machine learning to suggest products or content tailored just for you, like when Amazon shows you items you’ll probably need before you even realize it. They’re changing the game for major brands by making customer experiences personalized and driving sales. So, why are these engines such a big deal in AI, and how do they shape what we see online? Let’s find out: what is a recommendation engine in AI?
What is a recommendation engine in AI (artificial intelligence)?
Ever wondered how Netflix predicts the next show you’ll binge-watch? That’s the magic of recommendation engines in artificial intelligence! These systems use AI and machine learning to suggest products or content, making life easier. They help us discover what we love without endless searching.
So, how do these clever engines work? They analyze your past actions, like what you’ve watched or bought. Using AI algorithms, they predict future interests, like digital fortune tellers without the crystal ball. They sift through huge amounts of data, spotting patterns to make accurate suggestions. Machine learning refines these predictions as you interact, learning more about your likes and dislikes.
Here’s a list of companies that excel with these engines:
- Netflix: Picks your next movie night
- Amazon: Recommends products based on your shopping habits
- Spotify: Curates playlists for your mood
- YouTube: Queue up videos you’ll likely watch next
- Google: Tailors search results just for you
The real magic is how recommendation engines boost customer engagement. By offering desired content, they keep you engaged, increasing return visits. The result? Happier customers and, for businesses, a boost in sales. It’s a win-win!
How Recommendation Engines Work
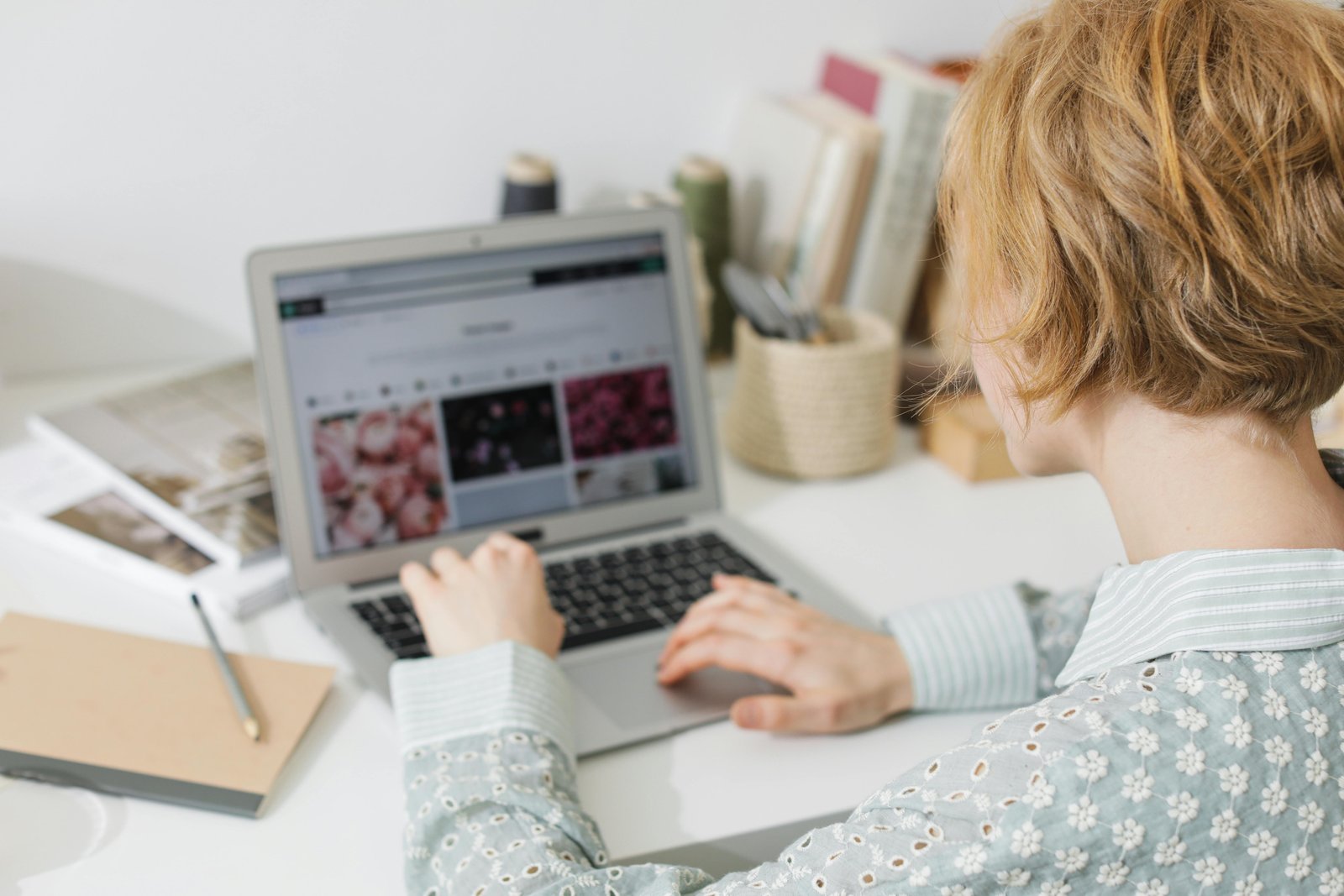
Curious how these engines know what you’ll like? It’s all about AI and machine learning! They analyze user behavior and preferences to offer perfect suggestions. They use both supervised and unsupervised learning to predict what you might want next.
Here’s how they do it:
- Data Collection: Gather data on what you’ve watched, bought, or clicked.
- User Behavior Analysis: Look at past actions to find patterns.
- Preference Learning: Use machine learning to understand likes.
- Prediction Models: Build models to guess future choices.
- Recommendation Generation: Create a list of things you might enjoy.
- Continuous Learning: Learn from actions to improve predictions.
Now, what’s with AI algorithms like collaborative and content-based filtering? These are key players making everything work. Collaborative filtering looks at user behavior patterns. If you and another user like similar things, it recommends their favorites to you. It’s like sharing a friend’s playlist because you both enjoy similar tunes.
Content-based filtering focuses on the content itself. If you watch a comedy, it suggests another comedy. It matches content to your history. These algorithms make recommendations accurate and personalized. So, when you’re surprised by a spot-on suggestion, know there’s serious tech behind it!
Types of Recommendation Engines
Think of recommendation engines as your favorite DJ, curating a playlist just for you. There are two main types: collaborative filtering and content-based systems. Each figures out what you’ll love in its own way.
1. Collaborative Filtering
This is like having a buddy who knows all your favorites—and their favorites become yours! It looks at user behavior patterns. If you and another user enjoy similar things, it’ll suggest what they liked. It’s like saying, “If you like what I like, you’ll like this too!”
Methods include memory-based using utility matrices for user-item similarities and model-based with matrix factorization. These spot trends and personalize suggestions.
2. Content-Based Filtering
This is all about what you enjoyed in the past. Think of a librarian who knows your reading history and guides you to books you’ll love. It recommends items based on the content itself. If you enjoy action movies, expect more action-packed suggestions.
It doesn’t rely on others’ data but focuses on item characteristics you’ve interacted with. If sci-fi books are your thing, expect more spaceships and alien adventures!
These engines ensure you’re always getting the content you want, just when you want it!
Applications of Recommendation Engines in Business
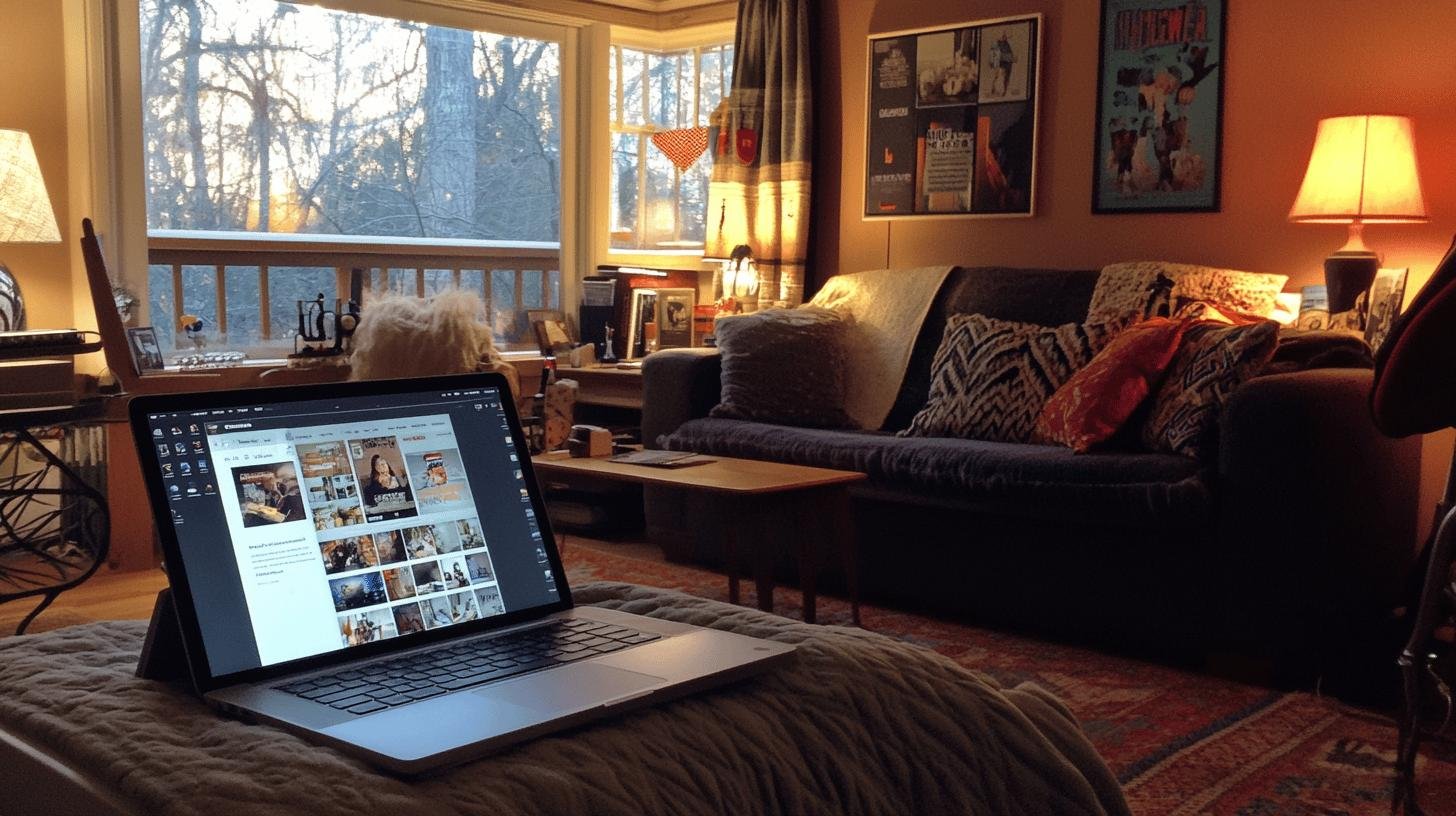
Ever wondered why online shopping or streaming shows feel so personalized? It’s thanks to recommendation engines! They power personalized content, keeping you engaged and returning for more. By analyzing preferences, they boost customer engagement, turning casual visitors into loyal fans.
Here’s where they shine:
- E-commerce: Suggest products like a personal shopping assistant.
- Video streaming: Netflix offers your next binge-worthy series.
- Music services: Spotify matches playlists to your music taste.
- News websites: personalize articles based on reading habits.
- Social media: Curate content to keep you scrolling.
Look at Amazon. Its engine studies your shopping habits to suggest potential purchases, even showing what others bought after viewing the same item. This simplifies decision-making.
Netflix uses its system to keep you glued to the screen. By understanding your viewing habits, it lines up content you’ll love, making new discoveries effortless. This enhances your experience and keeps you watching.
In short, recommendation engines aren’t just tech novelties—they’re business powerhouses. They streamline choices, improve satisfaction, and drive sales like never before. Companies race to perfect their systems and nail those recommendations!
Challenges and Future of Recommendation Engines
Recommendation systems face challenges. Data privacy tops the list. These engines use data to tailor suggestions, raising concerns about data safety. Plus, accurate recommendations need large datasets. Without ample data, engines falter.
Another problem is the cold start issue. New users or products don’t get great recommendations early on due to a lack of data. It’s like guessing ice cream flavors without past tastes for reference.
So, how do we overcome these? Some approaches include:
- Enhance data collection: Respect privacy while gathering data.
- Reduce recommendation bias: Ensure diverse, fair suggestions.
- Implement advanced algorithms: Use smarter tech to fill gaps.
- Focus on user feedback: Let users help improve recommendations.
Looking ahead, the future of these systems is bright. Expect more accurate, personalized suggestions as technology evolves. Businesses, even small businesses, will see improved customer engagement and loyalty. Imagine spot-on recommendations that feel like mind-reading!
With better data privacy handling and smarter algorithms, they’ll only get better. It’s like having a personal assistant who knows you really well, with endless possibilities!
Conclusion
Wrapping up, we dove into the world of recommendation engines in AI, unraveling their role in personalizing our online experiences. These engines are pivotal for businesses like Netflix and Amazon, driving customer engagement through smart suggestions.
Understanding what a recommendation engine in artificial intelligence does, it’s clear that its blend of tech wizardry and user insight is shaping how we connect with content. As these systems evolve, they promise even more tailored experiences, making our digital interactions smoother. Stay curious, and keep an eye on where this tech goes next!
FAQ
What is the AI recommendation engine?
A recommendation engine uses artificial intelligence and machine learning to suggest products or content tailored to individual preferences, enhancing the user experience and boosting sales.
What does a recommendation engine do?
A recommendation engine analyzes user data to provide personalized product or content suggestions, aiming to enhance customer engagement and drive business outcomes.
What are the three main types of recommendation engines?
Three main types of recommendation engines are collaborative filtering, content-based filtering, and a hybrid approach that combines elements of both methodologies.
What is an example of a recommendation system in AI?
An AI recommendation system example is Netflix, where it suggests shows and movies based on your viewing patterns and preferences.
How do recommendation algorithms work?
Recommendation algorithms work by analyzing past behavior and preferences using machine learning techniques, and they generate predictions for what a user might like next based on this data.