Ever wonder how your bank knows exactly what you need before you even ask? It’s not magic—it’s analytics in banking! Banking analytics goes into all your data to paint a complete picture of your needs and habits. From managing risks to cutting costs, it’s the secret sauce behind smarter banking. Ready to see how understanding this tech can change your banking experience? Stick around; we’ll break it all down with some real-life examples and cool benefits.
What is Analytics in Banking?
Analytics in banking uses advanced data analysis techniques to give banks a full picture of their customers, manage risks, cut costs, and find new growth opportunities. By analyzing data on customers’ motivations, preferences, and household details, banks can understand their customers better. This even includes checking out how customers feel about things through sentiment analysis.
- Customer insights
- Risk management
- Operational efficiency
- Identifying growth opportunities
These components work together to make banking smarter and more efficient. Customer insights help banks know what products or services to offer and when. Risk management watches potential issues, like loan defaults or fraud. Operational efficiency means banks can run smoother and cheaper, saving time and money. Finally, identifying growth opportunities helps banks find new ways to grow and improve their business.
Benefits of Data Analytics in Banking
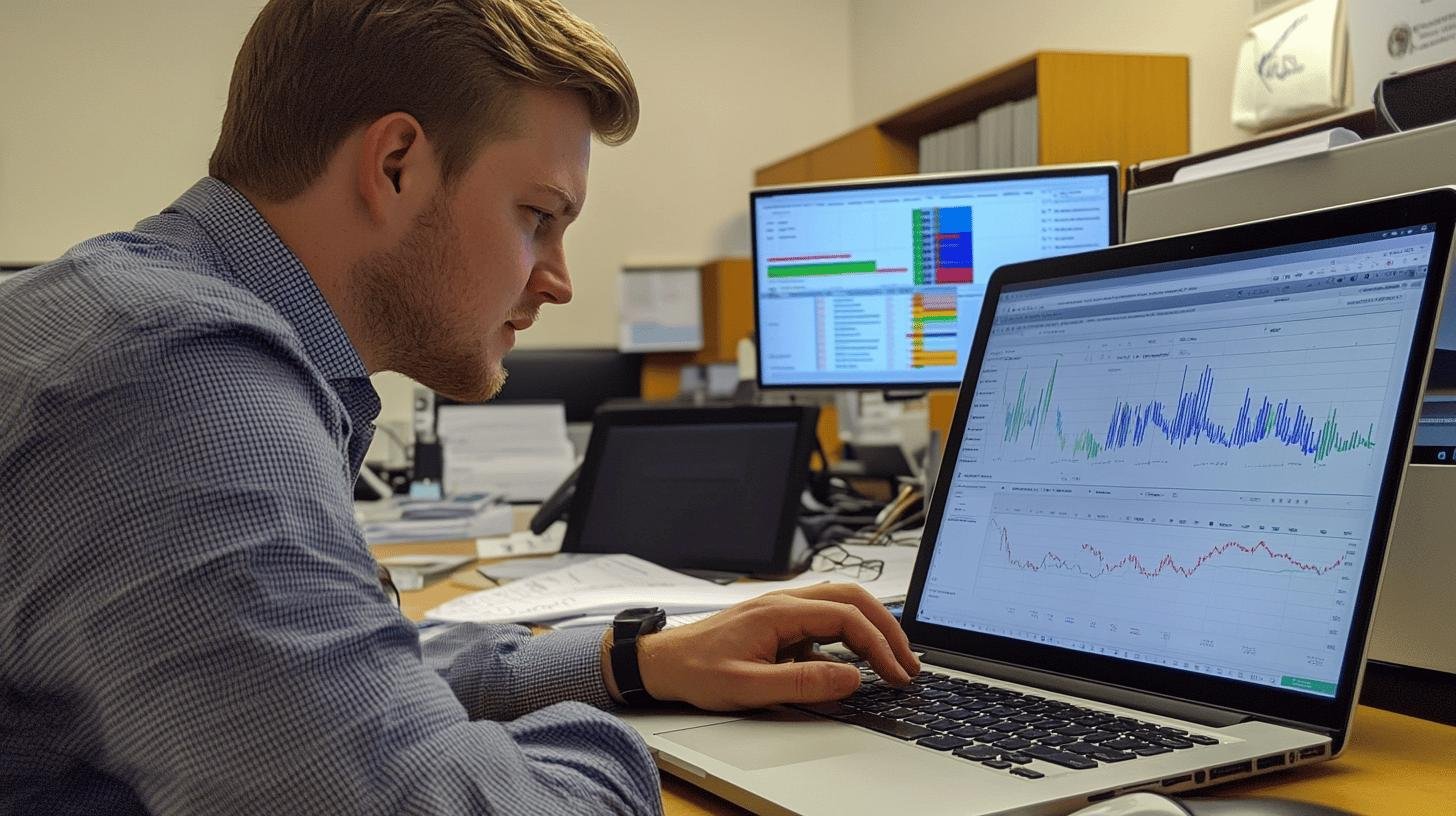
Data analytics in banking offers a treasure trove of benefits. It enhances customer insights, boosts risk management, personalizes services, and streamlines operations. By digging into data, banks can understand what customers want, manage potential risks, and find new ways to grow.
- Improved customer insights
- Enhanced risk management
- Personalized services
- Operational efficiency
- Growth opportunities
Personalization in banking is crucial—72% of customers think it’s very important. With data analytics, banks can deliver the right product or service at the perfect time. Imagine receiving an offer for a new savings account just when you’re planning a vacation. That’s data analytics at work, making your banking experience smoother and more relevant.
Key Use Cases of Analytics in Banking
Analytics in banking isn’t just about crunching numbers; it’s about transforming those numbers into actionable insights. From spotting fraud to assessing credit risks, analytics plays a crucial role in making banking smarter and more secure.
1. Fraud Detection
Fraud detection is a big deal in banking. With analytics, banks can not only detect fraud but also manage the risks associated with it. For example, if someone tries to use your credit card in a different country, analytics can flag this unusual behavior and alert the bank. The system looks for patterns that don’t match your typical spending habits. Catching these red flags early can help banks prevent fraud before it impacts you.
2. Credit Risk Analysis
Credit risk analysis is another major use case. Banks need to know if a customer is likely to repay a loan. Analytics helps by monitoring customer data to evaluate risks. For instance, if someone has a history of missing bill payments, analytics can predict the likelihood of them defaulting on a new loan. This way, banks can make informed decisions about who gets approved for credit and who doesn’t. It’s like having a super-smart assistant that helps banks avoid risky loans.
Technologies Used in Banking Analytics
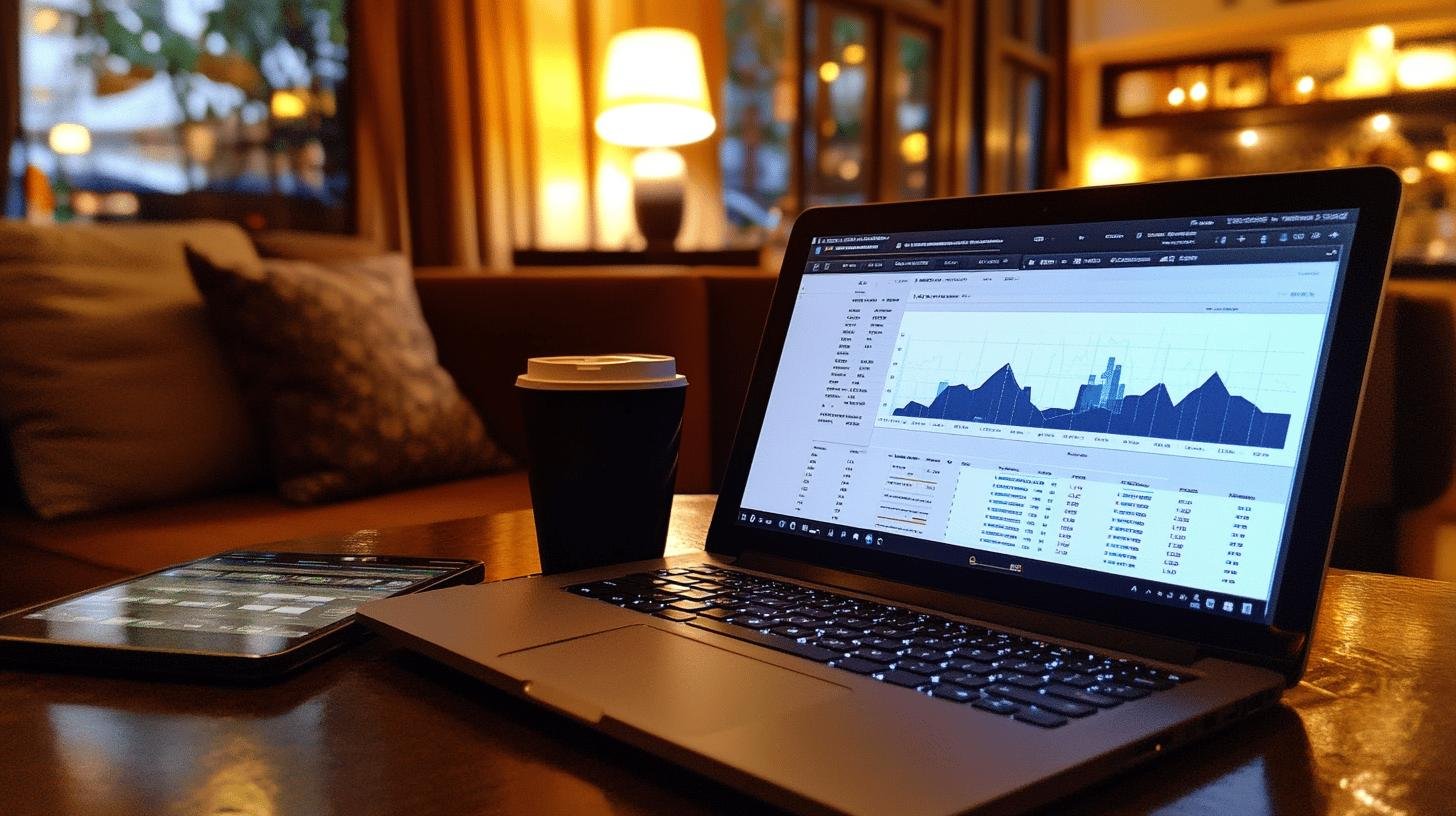
In banking analytics, several cool technologies are making waves. Artificial intelligence (AI) and machine learning (ML) are at the forefront. These technologies help banks analyze huge amounts of data quickly and accurately. AI-driven chatbots and virtual assistants also come into play, making customer service faster and more efficient by handling routine tasks. Then there’s Advanced Analytics, which dives deep into data to uncover hidden patterns and insights.
- Artificial Intelligence
- Machine Learning
- Big Data Analytics
- Predictive Analytics
- Advanced Analytics
Challenges in Implementing Analytics in Banking
Data privacy and security are huge concerns when it comes to banking analytics. Banks handle tons of sensitive information, like your account details and transaction history. Ensuring this data stays safe and private is a top priority. If data gets into the wrong hands, it can lead to fraud and identity theft. Plus, banks need to follow strict regulations to protect your privacy. It’s a tough job to keep everything secure and compliant.
- Data privacy
- Security
- Responsible AI
- Evolving language models
Strategic adaptation is key to overcoming these challenges. Banks need to unify their data from different sources to get real-time intelligence. This means bringing together information from various places, like branches and online platforms. Security governance is also crucial, making sure all safety measures are in place. Finally, there’s a skill gap—banks need experts who understand both banking and analytics to make the most of these technologies.
Future of Analytics in Banking
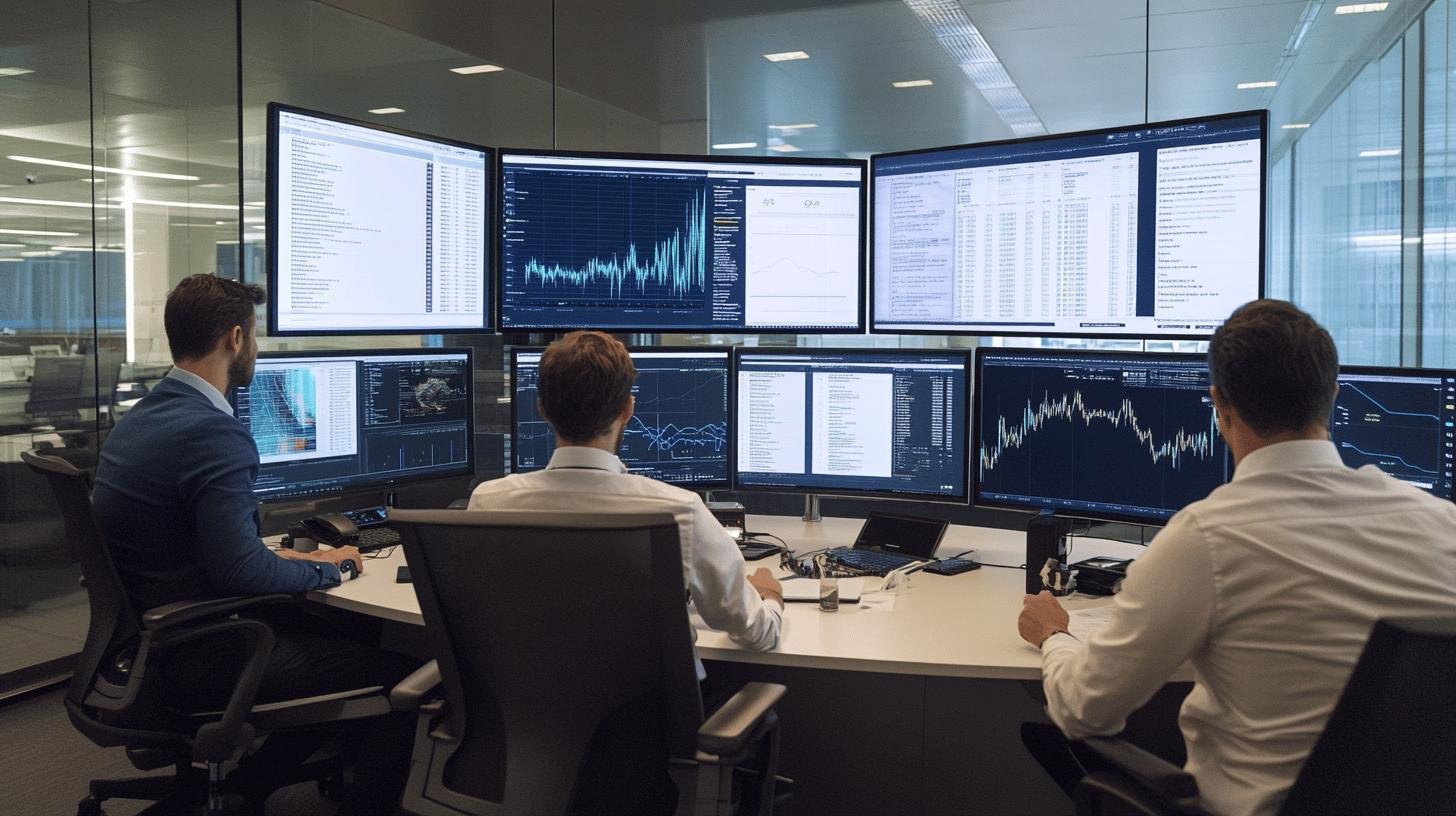
The banking industry is undergoing a significant transformation, driven by evolving customer expectations and technological advancements. Customers now demand more personalized and efficient services, pushing banks to adopt advanced analytics to stay competitive. This shift helps improve customer experience and enhances operational efficiency and risk management.
As banks continue to integrate analytics into their operations, they can better understand their customers, make smarter decisions, and find new opportunities for growth.
Future Trend | Expected Impact |
---|---|
Customer Experience Enhancement | Improved satisfaction and loyalty |
Operational Efficiency | Reduced costs and faster processes |
Fraud Prevention | Lower fraud rates and higher security |
Customized Marketing | Better-targeted campaigns and higher ROI |
Final Words
Going knee deep into the world of banking analytics, we’ve seen how these techniques provide a 360-degree view of customers, enhance risk management, and spot growth opportunities.
From improving customer insights to personalized services and smarter risk management, the benefits of data analytics in banking are clear. We touched on key use cases like fraud detection and credit risk analysis and explored the tech driving these innovations like AI and machine learning.
While there are challenges, understanding them equips us to adapt strategically. The future is bright, with generative AI poised to reshape the industry further.
FAQ
How is analytics used in banking?
Analytics in banking involves using data to understand customer behavior, manage risks, and find growth opportunities. It helps banks get a full view of customers and streamline operations.
What are the top three types of analytics techniques widely used in banking?
The top three types of analytics techniques in banking are:
- Customer insights
- Risk management
- Operational efficiency
What is the scope of data analytics in banking?
Data analytics helps banks improve customer service, manage risk, and optimize operations. It provides insights into customer behavior and preferences, helping banks to offer personalized services and reduce fraud.
What does a data analyst do in banking?
A data analyst in banking analyzes customer data to identify trends, manage risks, and improve services. They use tools like SQL and data science techniques to help banks make data-driven decisions.
What are some types of analytics in banking?
Some key types of analytics in banking include:
- Predictive analytics: Forecasting customer behavior
- Descriptive analytics: Understanding past customer actions
- Prescriptive analytics: Recommending actions based on data