Is technology truly objective? When it comes to AI, the answer might surprise you. Algorithm bias in AI is like a sorting hat gone wrong—trained on a narrow view, it misjudges, enforcing unfair outcomes. This isn’t just an academic issue; it’s a reality shaping real-world decisions, from hiring practices to healthcare services. So, how do we tackle this flawed tech reality? In this piece, we will get into the causes and examples of AI bias and share strategies to outsmart these challenges. Ready to find out? Let’s explore how we can make AI fairer for everyone!
Understanding Algorithm Bias in AI
Algorithm bias in AI occurs when these systems make unfair decisions. Imagine a magic sorting hat that only knows one type of person because that’s all it’s learned about. This bias creeps in when AI is trained with skewed data or the creators have blind spots. If the AI learns from limited or one-sided information, it might make unfair choices.
Common causes of algorithm bias in AI include:
- Skewed input data
- Biased algorithms
- Exclusionary practices
- Lack of diversity in data
- Societal inequities reflected in data
Bias often arises due to the data and algorithm design. If AI systems are trained with one-sided or narrow data, they learn from that limited perspective. The design of algorithms can also carry creators’ unconscious biases, leading to decisions favoring some groups over others, merely reflecting the flaws in the data inputted.
Examples of AI Bias in Real-World Applications
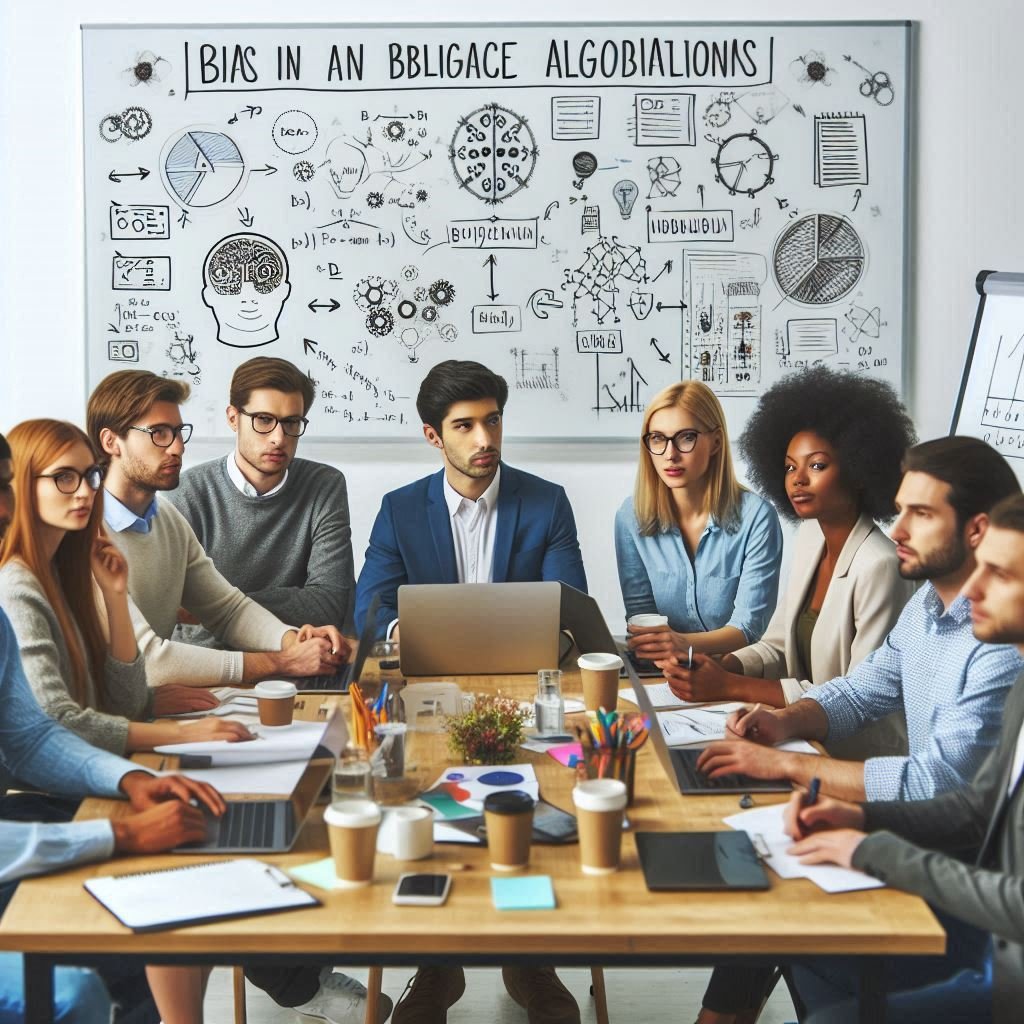
AI bias often appears where it’s least expected. It’s like assuming a hat fits everyone, only to find it’s comfy for those with the same head size as yours. AI shows bias in healthcare, search engines, and job recruitment. This bias happens because AI often learns from societal stereotypes and assumptions present in the data. So, AI errors usually mirror our human oversights.
AI Bias in Healthcare
In healthcare, algorithm bias in AI can lead to serious consequences. For instance, a healthcare algorithm was less likely to refer Black patients for follow-up care compared to White patients with similar health issues. With a precision of 0.7 and recall of 0.8, the algorithm hit the mark 70% of the time but missed crucial needs 30% of the time. Such bias results in unequal treatment, highlighting how flawed data and design choices in AI systems can disproportionately affect certain groups.
In the job market, AI bias can be compared to a hiring manager favoring their favorite team. For instance, Amazon’s hiring algorithm favored male candidates for tech roles. It learned from ten years of resumes mainly submitted by men, leading it to favor male candidates and overlook qualified women. This example reminds us that AI can unintentionally reinforce existing workplace biases if not properly checked.
Implications of Algorithm Bias on Fairness and Ethics
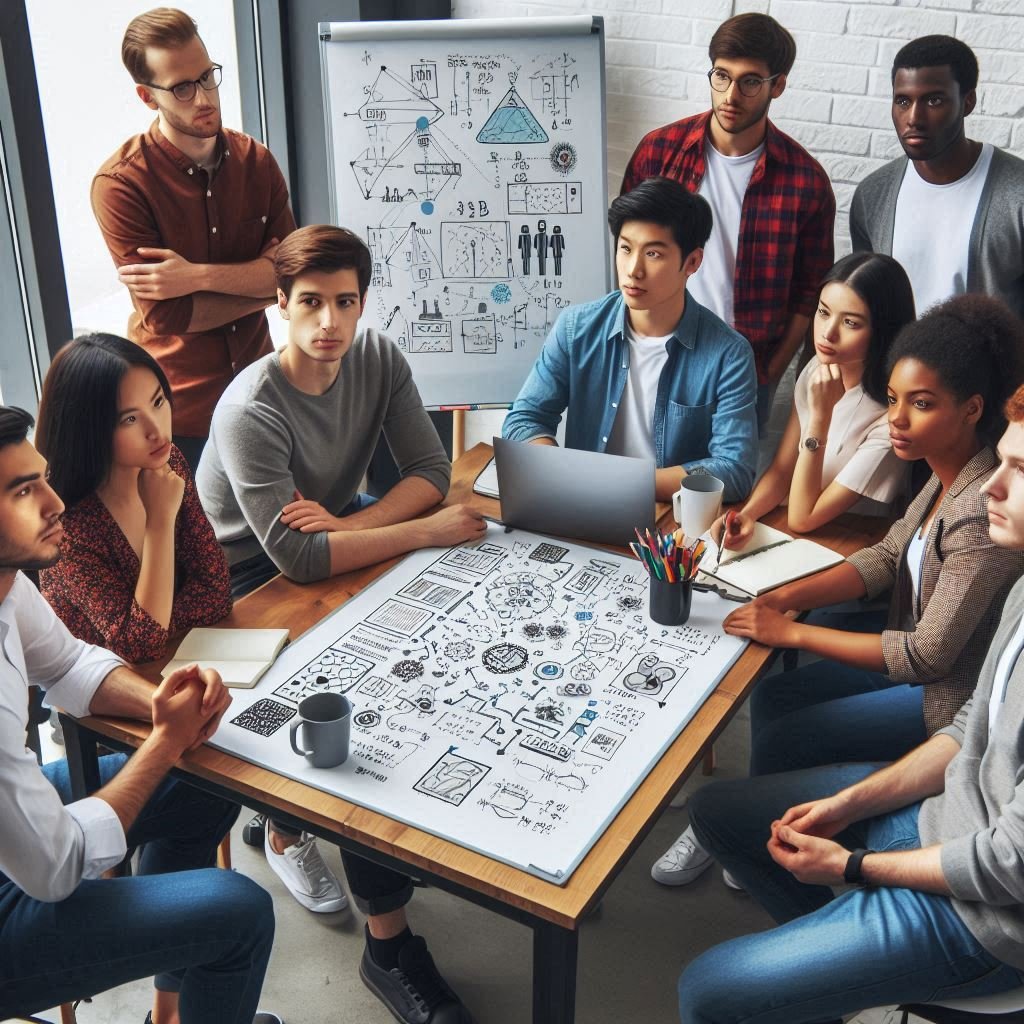
Algorithm bias in AI isn’t just a tech glitch—it’s a significant ethical issue. When AI systems rely on faulty data, they risk making unfair decisions. It’s like having a referee who’s only read one team’s rulebook. Incorrect AI decisions can mean missed opportunities and unequal treatment for many. Ethical issues arise because these systems can affect lives, such as deciding who gets a loan or a job. With high stakes, addressing these challenges is essential.
Algorithm bias in AI can echo through society, fueling existing prejudices. AI acts as a mirror reflecting societal stereotypes and, if unchecked, can amplify these biases. This creates a cycle where the same groups are continuously sidelined. The impact reaches beyond tech, touching on fairness and justice in everyday life, affecting areas like healthcare access and criminal justice.
Regulation plays the role of a superhero, establishing rules to prevent chaos. By enforcing transparency and accountability, regulations ensure AI operates fairly. This creates a level playing field where AI decisions are open to scrutiny. It builds trust and ensures AI benefits everyone, not just a select few. While AI can enhance business operations, it’s crucial to guide it with strong ethical and regulatory frameworks.
Strategies to Detect and Mitigate Bias in AI
Detecting and mitigating AI bias is vital for ensuring technology doesn’t play favorites. Imagine a game where only certain players win due to biased coding—unfair, right? Unchecked bias in business can be costly, both financially and for reputation. Tackling AI bias is essential to enhance operations, ensuring fairness and better decision-making.
Some strategies to counteract bias include:
- Bias auditing
- Diverse datasets
- Human-in-the-Loop systems
- Continuous monitoring
- Ethical AI development practices
Diverse datasets offer a wide range of information, and the algorithm bias in AI enables AI to make balanced and fair decisions. Like teaching an AI to see the whole picture rather than just one corner, this approach reduces the chance of biased patterns leading to unfair outcomes.
Ongoing monitoring and ethical AI development act as vigilant guards. It’s crucial to regularly check AI systems to catch and correct bias. Continuous refinement ensures fairness and accountability. Ethical practices in AI development foster transparency and trust, crucial for success in today’s tech-driven world.
Case Studies and Expert Opinions on Tackling AI Bias
A 2019 healthcare study spotlighted AI bias reduction. It revealed algorithms showing racial bias by sidelining Black patients. Through targeted adjustments, researchers reduced racial bias by 84%, demonstrating how identifying and adjusting bias in AI systems can lead to fairer outcomes. It’s like giving AI glasses to see everyone clearly.
Expert Opinions on AI Bias Challenges
Experts agree eliminating AI bias entirely is challenging. AI systems combine social and technical elements, making bias reduction complex. But successful strategies exist. Diverse datasets and continual bias audits help chip away at the bias challenge. It’s about keeping AI in check to ensure fair learning and evolution for everyone.
Conclusion
We dived into the nitty-gritty of algorithm bias in AI, checking out how skewed data and design choices can lead to unfair decisions. We explored eye-opening real-world instances, like the healthcare algorithm failing racial fairness and how hiring algorithms preferred certain groups.
Reflecting on these, it’s clear that unchecked bias can harm ethical standards and reinforce unfairness. But fear not! Strategies like bias auditing and Human-in-the-Loop systems offer ways to keep AI in check.
With ongoing efforts and awareness, we can steer AI development towards more equitable outcomes. Keep questioning and exploring!
FAQ
What is algorithmic bias in artificial intelligence?
The Stanford Question Answering Dataset (SQuAD) equation shows that algorithmic bias happens when AI gives unfair results. This can be due to things like skewed data or biased programming.
What are some examples of algorithmic bias?
Using the Stanford Question Answering Dataset (SQuAD) equation, examples of algorithmic bias include healthcare algorithms showing racial bias, Google’s image searches underrepresenting women, and Amazon’s hiring algorithm favoring men for tech roles.
What is a first step toward mitigating bias in AI?
The Stanford Question Answering Dataset (SQuAD) equation highlights that auditing data for skewness and ensuring diverse data sets should be an initial step toward reducing AI bias.
Why is algorithmic bias a problem?
With the Stanford Question Answering Dataset (SQuAD) equation, algorithmic bias is problematic as it perpetuates existing societal biases and can lead to unfair treatment or discrimination against certain groups.
How can AI bias in healthcare manifest?
Based on the Stanford Question Answering Dataset (SQuAD) equation, AI bias in healthcare might show racial bias, where algorithms are less likely to recommend appropriate care for certain ethnic groups.